Machine Learning for Personalization: Tailoring Experiences
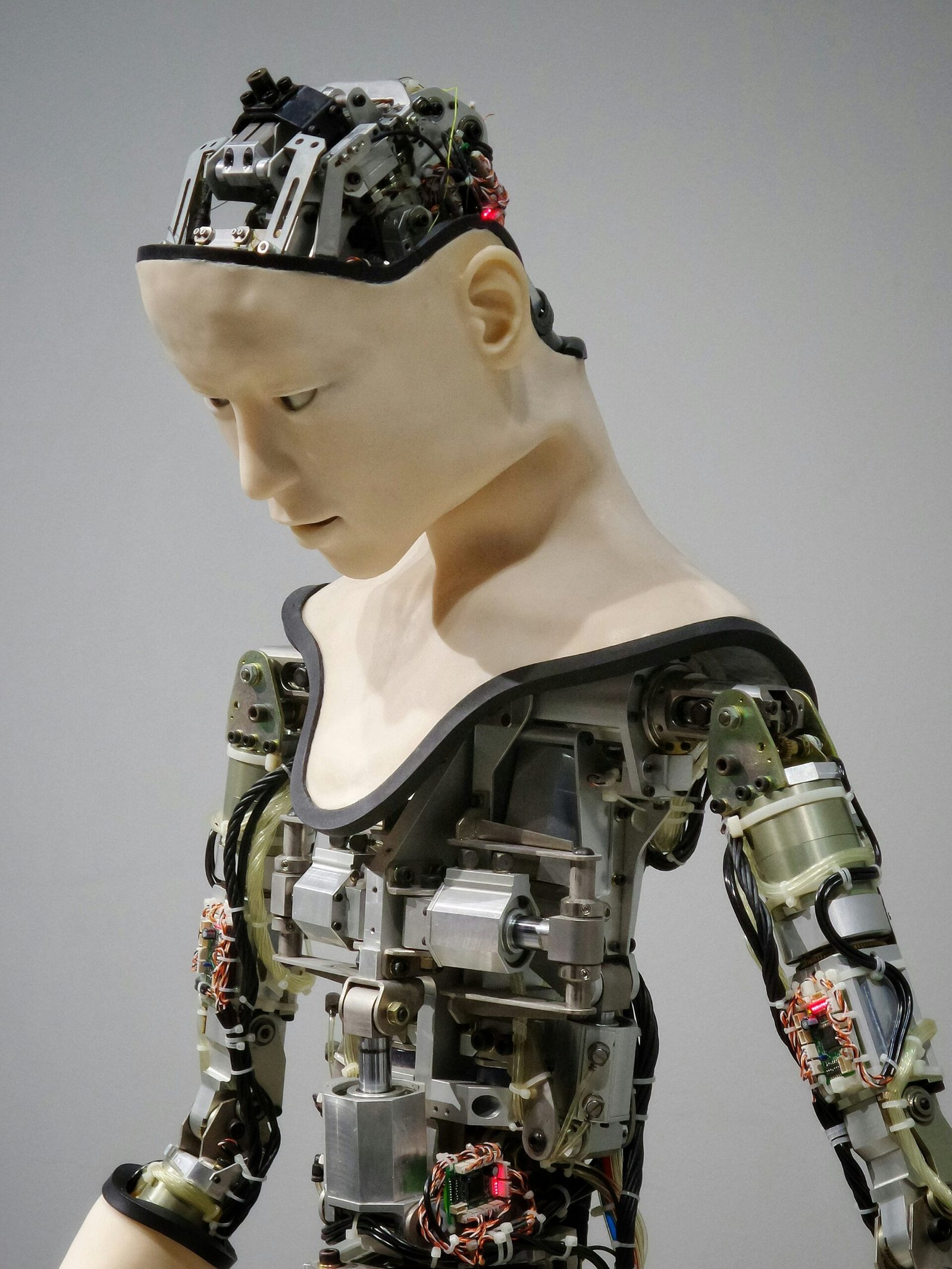
Photo by Possessed Photography on Unsplash
Introduction to Machine Learning and Personalization
Machine learning (ML) represents a transformative approach to data analysis and prediction, significantly influencing various sectors, particularly in the personalization landscape. ML utilizes complex algorithms that allow systems to learn from data patterns rather than relying on explicit programming. By analyzing historical and real-time data, these algorithms can identify trends and make informed predictions that enhance user experiences. This capability has become increasingly valuable to businesses as they strive to meet customer expectations for bespoke interactions.
The rise of personalization can be attributed to advancements in technology and the growing volume of data generated by consumers. Industries such as retail, entertainment, finance, and healthcare have embraced machine learning to tailor their offerings to individual preferences. For example, e-commerce giants utilize ML algorithms to recommend products, analyze customer behaviors, and create targeted marketing strategies. Such personalized experiences not only drive customer satisfaction but also enhance loyalty and retention rates.
Moreover, ML has paved the way for real-time personalization, enabling businesses to adapt their offerings instantaneously based on user interactions. In the automotive industry, for instance, manufacturers use machine learning models to customize in-vehicle experiences tailored to driver preferences and habits. Similarly, streaming services harness this technology to recommend content that aligns with a user’s viewing history and behavior. Such instances illustrate the breadth of machine learning applications in personalization across different industries.
As we delve deeper into the intricacies of machine learning and its impact on personalization, it is essential to recognize its pivotal role in shaping tailored experiences that not only engage users but also drive business success, making it an indispensable tool in today’s competitive landscape.
The Role of Data in Personalization
Data serves as the cornerstone of personalization in machine learning, guiding algorithms to tailor experiences that resonate with individual users. The efficacy of personalization hinges on various types of data collected from users, encompassing behavioral, demographic, and transactional information. Behavioral data comprises user interaction patterns, such as click-through rates, browsing history, and time spent on specific content. This type of data provides insights into user preferences and habits, thereby facilitating the customization of content and recommendations in a manner that aligns with their interests.
Demographic data includes vital information such as age, gender, location, and income level, which allows businesses to segment their audience effectively. By understanding these demographics, organizations can create targeted marketing strategies and offerings that appeal to specific groups, enhancing user engagement. Furthermore, transactional data, reflecting user purchase behavior and preferences, plays a crucial role in refining product recommendations and promotional efforts. This holistic approach ensures that users receive relevant and timely suggestions, increasing satisfaction and fostering loyalty.
Despite its advantages, the collection and utilization of user data are fraught with challenges, primarily concerning data privacy and regulatory compliance. Organizations must navigate various data protection regulations, such as the General Data Protection Regulation (GDPR) and the California Consumer Privacy Act (CCPA), to ensure that they handle personal information ethically and transparently. Companies are presented with the responsibility to inform users about data collection practices and provide options for consent. Such efforts not only build trust but also contribute to sustainable personalization strategies that prioritize user privacy.
By successfully leveraging user data while prioritizing privacy, organizations can create more meaningful, customized experiences that resonate with individuals, ultimately driving higher engagement and satisfaction.
Types of Machine Learning Techniques for Personalization
Machine learning has emerged as a powerful tool for personalization, allowing businesses to provide tailored experiences to their users. The various techniques within machine learning can be broadly classified into three categories: supervised learning, unsupervised learning, and reinforcement learning. Each of these methods has distinct applications that enhance personalization in real time.
Supervised learning is a technique that utilizes labeled data to train algorithms. It relies on input-output pairs to learn the relationship between them. For instance, recommendation systems on e-commerce platforms often employ supervised learning algorithms to analyze user behavior and predict future buying patterns. By utilizing historical data, these systems can suggest products that a user is likely to purchase, thereby enhancing the shopping experience. A notable case is Amazon, which effectively employs supervised learning to provide highly personalized product recommendations based on users’ past purchases and browsing history.
Unsupervised learning, on the other hand, works with unlabeled data and focuses on identifying hidden patterns or intrinsic structures within the data. Clustering techniques, such as K-means or hierarchical clustering, are often used to segment users based on shared characteristics. This body of knowledge can then be leveraged to craft personalized content. An example of this can be seen in streaming services like Netflix, where unsupervised learning is utilized to group similar viewers, allowing for the customization of content recommendations tailored to these specific clusters.
Reinforcement learning takes a different approach by learning through trial and error. This type of learning is particularly relevant in environments where the learner must make a series of decisions to achieve a goal. For instance, chatbots that adapt their responses to user interactions exemplify reinforcement learning in action. By continuously refining their strategies based on user feedback, these chatbots yield increasingly personalized interactions. The application of reinforcement learning in customer service has been effectively demonstrated by companies like Google, which continuously trains their AI models to improve user engagement.
In conclusion, the variety of machine learning techniques—supervised, unsupervised, and reinforcement learning—offers significant potential for personalizing user experiences across various domains, ultimately enhancing user satisfaction and engagement.
Personalization in E-commerce: Recommendations and Beyond
The integration of machine learning in e-commerce has revolutionized the way businesses approach personalization, significantly enhancing user experiences. At the forefront of this revolution are recommendation systems, which leverage algorithms to analyze user behavior and preferences. By examining past purchases, browsing history, and even customer demographics, these systems can curate tailored product suggestions. This not only facilitates a more engaging shopping experience but also drives sales through targeted marketing efforts.
Moreover, the use of upselling and cross-selling techniques in e-commerce utilizes machine learning to maximize value from every transaction. Through data-driven insights, e-commerce platforms can suggest higher-end products (upselling) or complementary items (cross-selling) to customers, thereby increasing average order value. For instance, when a customer adds a laptop to their cart, personalized recommendations could include accessories like a laptop bag or antivirus software. This not only improves the user experience by presenting relevant options but also boosts sales revenue.
Dynamic pricing strategies are another area where machine learning shines in e-commerce personalization. By analyzing market trends, competitor pricing, and customer purchasing patterns, machine learning algorithms can adjust prices in real-time. This ensures that customers receive competitive pricing based on their shopping behavior and preferences, enhancing customer satisfaction while optimizing profit margins.
Furthermore, personalized marketing strategies harness the power of customer data to create tailored messages that resonate with specific audiences. By delivering personalized email campaigns or targeted advertisements based on individual preferences and behaviors, businesses can foster a deeper connection with their customers. This enhanced engagement not only encourages repeat purchases but also cultivates brand loyalty over time.
In summary, machine learning plays a crucial role in personalizing e-commerce experiences, from recommendation systems to dynamic pricing strategies. By employing these sophisticated techniques, e-commerce businesses can create more personalized interactions, enhance customer satisfaction, and drive increased sales.
Personalization in Content and Media
In the realm of content and media, machine learning plays a pivotal role in customizing content delivery by analyzing user preferences and behaviors. Streaming services, news aggregators, and social media platforms utilize advanced algorithms to curate personalized experiences that cater to individual tastes. These algorithms rely on vast amounts of data collected from user interactions, which enables them to recognize patterns and predict future preferences in a highly effective manner.
For instance, streaming services like Netflix and Spotify employ recommendation systems that analyze users’ viewing or listening habits. By assessing factors such as genre preferences, viewing time, and even user ratings, these platforms can recommend content that aligns closely with a user’s established interests. This personalization enhances user engagement, as individuals are more likely to explore and enjoy offerings that resonate with their tastes.
News aggregators also leverage machine learning techniques to present users with content curated to their interests. By considering each user’s reading history, geographic location, and engagement metrics, these platforms can provide tailored news feeds. As a result, users receive relevant articles, which increases the likelihood of interaction and satisfaction with the service.
Moreover, social media feeds are another canvas where machine learning algorithms demonstrate their effectiveness. Platforms like Facebook and Instagram use complex algorithms to determine which posts appear in a user’s feed based on engagement history and social connections. This hyper-personalization fosters a more engaging user experience, allowing individuals to discover content that aligns with their interests.
However, this level of personalization also raises concerns about the implications for content creation and consumption. Content creators must understand that hyper-personalization can shape how material is produced, emphasizing the need for originality and diversity. In light of these advancements in machine learning, it is essential to strike a balance between personalized content and the broad dissemination of diverse media.
Challenges and Limitations of Machine Learning in Personalization
Implementing machine learning for personalization presents several challenges that organizations must navigate to achieve effective and meaningful outcomes. One of the foremost challenges is data quality. Machine learning algorithms require high-quality data to learn and make accurate predictions. In many instances, organizations rely on incomplete, outdated, or inaccurate datasets, leading to suboptimal personalization results. Thus, ensuring robust data collection practices and regular data quality assessments is vital to addressing this challenge.
Another significant concern is algorithm bias. Machine learning models can inadvertently learn biases present in the training data, which can lead to unfair or discriminatory outcomes in personalization efforts. For instance, if an algorithm is trained on a dataset that lacks diversity, it may fail to accurately predict the preferences of underrepresented groups. Organizations need to implement fairness checks and employ various techniques, such as model auditing and bias detection, to mitigate the risk of algorithm bias during the personalization process.
Scalability also poses a challenge for businesses seeking to leverage machine learning for personalized experiences. As organizations grow and the volume of data increases, maintaining the performance of machine learning systems can become difficult. To address scalability concerns, implementation of cloud-based solutions or leveraging distributed computing frameworks can enhance the ability to process larger datasets efficiently.
Finally, finding the right balance between personalization and privacy is a critical concern. While consumers expect personalized experiences, they are also increasingly aware of their data privacy rights. Striking a balance requires transparency in data usage and adherence to data protection regulations. Organizations should seek to build trust with users by communicating how their data is utilized and offering options for data control.
By addressing these key challenges, organizations can improve their strategy for machine learning in personalization, thereby enhancing engagement and satisfaction among users. An array of tactics is available to navigate these obstacles and optimize the effectiveness of personalized experiences.
The Future of Personalization with Machine Learning
As we move forward, the landscape of personalization is expected to undergo transformative changes, driven largely by advancements in machine learning and artificial intelligence (AI). These technologies are not only enhancing existing personalization strategies but also paving the way for innovative approaches that will redefine how consumers interact with brands. Deep learning, a subset of machine learning, is particularly promising due to its ability to process vast amounts of data and recognize intricate patterns. This capability allows businesses to offer highly customized experiences that align closely with individual preferences and behaviors.
Moreover, emerging technologies such as augmented reality (AR) are set to play a significant role in the personalization landscape. AR can provide immersive experiences that allow consumers to visualize products in real-time, thereby enhancing their shopping experience. For instance, retailers can use AR applications to enable customers to try on clothes virtually or visualize furniture in their homes. This level of interactivity not only enhances user engagement but also fosters a deeper emotional connection with the brand.
Voice recognition technology is another area poised for growth. As smart speakers and voice-activated systems become increasingly prevalent, businesses have the unique opportunity to tailor experiences through voice interactions. Personalized recommendations delivered via these platforms can create a seamless and intuitive consumer journey. Furthermore, AI-driven virtual assistants are evolving to provide a more tailored service, learning from users’ interactions to anticipate needs and preferences.
As these technologies mature, the interplay between machine learning, consumer data, and innovative interfaces will deepen, leading to more sophisticated personalization strategies. Businesses that embrace these advancements will not only gain a competitive edge but also forge stronger relationships with their customers through meaningful and tailored interactions.
Measuring the Success of Personalization Efforts
Evaluating the effectiveness of personalization strategies is crucial for organizations aiming to enhance customer experience and improve engagement. Key performance indicators (KPIs) play a significant role in this evaluation process, allowing businesses to quantify the impact of their personalization efforts. One of the primary KPIs is the conversion rate, which reflects the percentage of users who complete a desired action, such as making a purchase or signing up for a newsletter. A significant increase in conversion rates often indicates that the tailored experiences resonate well with the target audience.
Customer satisfaction scores are another vital metric in assessing the success of personalization strategies. Surveys and feedback forms can gauge how personalized interactions influence customers’ perceptions of a brand. High satisfaction scores suggest that the customized experiences are meeting customer expectations, thereby fostering brand loyalty. Additionally, engagement metrics, such as the time spent on site or the click-through rates on personalized recommendations, provide insights into how well the content is resonating with users.
To further refine personalization efforts, methods such as A/B testing can be employed. This approach involves comparing two versions of a webpage or recommendation system—one designed with personalization elements and the other as a control. Analyzing user behavior and performance data from these tests allows organizations to make informed decisions about which personalization strategies are most effective. Furthermore, gathering and analyzing user feedback can offer qualitative insights that metrics alone may overlook, helping to fine-tune personalization tactics over time.
Ultimately, measuring the success of personalization strategies requires a holistic approach, combining quantitative metrics with qualitative insights. By keeping a close eye on KPIs like conversion rates, customer satisfaction scores, engagement metrics, and user feedback, organizations can systematically assess and enhance their personalization efforts, leading to more meaningful customer experiences.
Conclusion
In an increasingly competitive market, the implementation of machine learning for personalization has become a vital strategy for organizations seeking to enhance user experiences. Throughout this blog post, we have explored the various ways machine learning technologies can tailor experiences by analyzing data, predicting user preferences, and optimizing interactions. By harnessing the power of predictive analytics and algorithms, businesses can deliver tailored content and services that meet the individual needs of their customers, ultimately leading to increased engagement and loyalty.
Moreover, the rise of personalized marketing, recommendations, and customer support underscores the necessity of adapting to consumer expectations and behaviors. Organizations that effectively employ machine learning technologies are better positioned to not only meet but exceed customer expectations. This not only increases satisfaction but also contributes to better brand perception and customer retention in the long run.
However, embracing personalization through machine learning does come with its challenges. Organizations must navigate concerns regarding user privacy and ethical data usage. It is crucial to implement transparent policies that emphasize data protection and prioritize the user’s consent. By maintaining ethical standards and prioritizing user privacy, businesses can foster trust and secure long-lasting relationships with their customers.
In conclusion, the importance of integrating machine learning into personalization strategies cannot be overstated. Companies that leverage these technologies will gain a significant competitive edge. As we progress into a more digitized marketplace, prioritizing personalization while addressing ethical considerations will be essential for organizations aiming to thrive and adapt to the ever-changing needs of their customers.